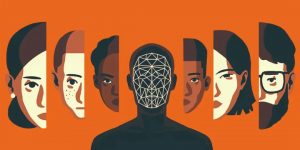
Is AI testing necessarily fair? Most people’s answer to this question is likely to be yes. In 2018, American social media Facebook developed a new AI system codenamed Rosetta to detect hate speech on its social platform. However, two years later, researchers at the University of Southern California found that all AI language detection systems, including Facebook, are actually biased, and messages sent by blacks, homosexuals and transgender people are more likely to be labeled “hate” by these AI. For example, black twitter (another social media in the United States, similar to China’s microblog) is 1.5 times more likely to be marked as “racist” by AI than other ethnic groups, and this value is even as high as 2.2 times in other studies.
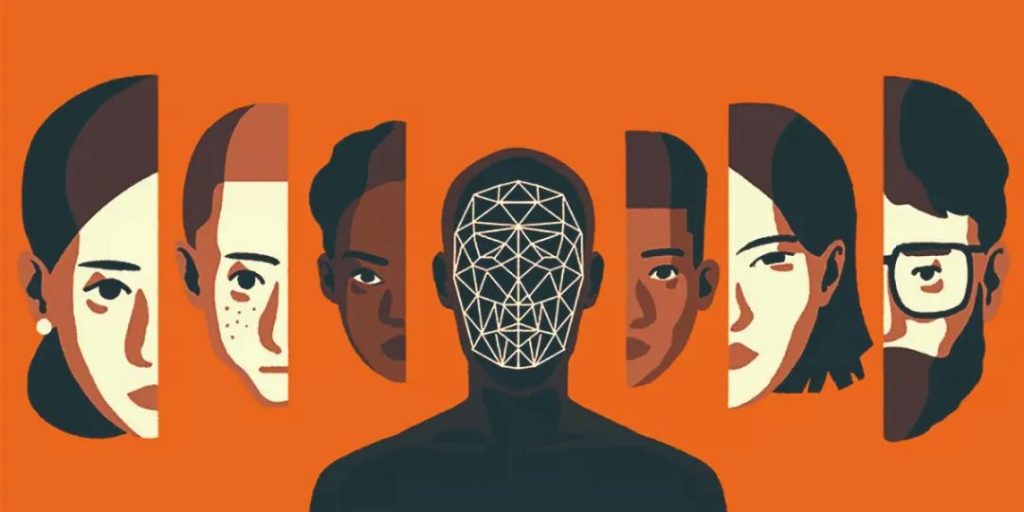
AI is not as fair as we think. What’s the matter?
Ai of “learning bad”
Why is AI biased? This is because AI is “learning bad”. AI is developed on the basis of a certain machine learning model, and all machine learning models need a lot of data to train and enrich their own database. If AI is compared to a high-rise building, the machine learning model is the design drawing of the AI high-rise building, and those data are the bricks and tiles used to build the AI high-rise building.
However, the data used in the machine learning model come from major social platforms in real life. The information in these social platforms is full of bias, and some social platforms even serve racists. Therefore, it is not surprising that AI tall buildings built on “Prejudice” bricks and tiles are biased.
In addition, these AI still use the detection method of “keyword”, completely ignoring the context and context. Take the English word “nigger”, which means “nigger” in Chinese. It is a word with racial discrimination and one of the key words detected by AI. However, if the situation is that blacks themselves say the word nigger (no matter who the object is), the word means “good brother”, “good friend” or “dead ghost” (dead Ghost: in the context of black women calling their black husband), and so on. In daily spoken English, blacks also use nigger to call their close friends and brothers.
But AI can’t control so much. As long as nigger or other similar words or statements appear in the message, the message will be marked by AI and then shut down in a “small black room”. At the same time, AI will also record the “crime” of the user who sent the message. As a result, as mentioned at the beginning of the article, black Twitter is more likely to be marked as “racist”.
AI in context is fairer
So how can scientists improve AI to make its detection of hate speech more fair? The first method that appears in our mind is probably to solve the problem of “brick and tile”. Since one of the reasons why AI is biased is that the data it trains and uses are biased, wouldn’t it be good to provide AI with objective and fair data? However, the data from real life are more or less biased. If the absolute objective and fair data are manually produced, the workload is very huge, even too large to be realized.
Researchers at the University of Southern California programmed the original AI algorithm so that it can contact the context and judge whether there is insulting language in the context while identifying keywords or keywords. In other words, compared with the original AI, the programmed AI only considers two more “situations”.
What is the effect of the improved AI? Compared with other newly developed AI, even though the data used by researchers at the University of Southern California in the improved AI practice are all from the notorious hate website, it still has a higher accuracy of detecting hate speech, up to 90%, compared with 77% of other latest AI. Why did USC AI improve so much by considering two additional factors?
The reason behind it is not difficult to understand. The same and simple sentence “thank you, my nigger.” Thank you, my good brother, If we consider the context like the University of Southern California AI, we can easily understand that this sentence means thank you. However, if we ignore the context and only look at the keyword “nigger” like traditional AI, we will think that the speaker is making racist remarks.
Comments