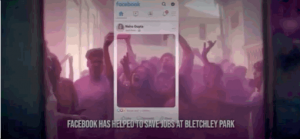
Yesterday, Facebook announced that its latest neural machine translation model can translate between 100 languages without relying on English, and called it a “milestone” progress. Some netizens posted questions today, saying that the statement of “milestone” is a bit exaggerated, and “not relying on English” is not accurate enough. There is no mention of Google’s research in this field.
Facebook lying again?
Yesterday, Facebook just announced that its machine translation has achieved milestone progress. It can achieve mutual translation between 100 languages and does not rely on the “intermediary” of English. Today, reddit netizens are here to lift the car.
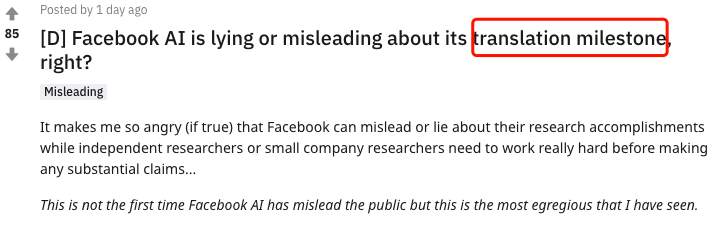
The netizen said that Facebook had previously exaggerated propaganda, but this time it was a bit too much.
Facebook’s translation between 100 languages is not a milestone?
Facebook claims that the latest model can perform machine translation in up to 100 languages directly, such as from Chinese to French, and does not require English as an intermediary during training. In evaluating the BLEU index, which is widely used in machine translation, it is 10% higher than the performance of English-centric translation systems.
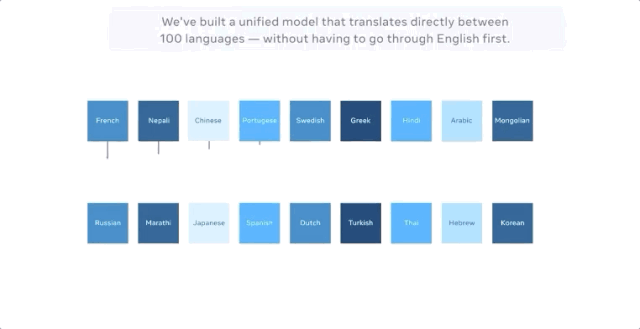
The Facebook AI Lab blog did not mention that Google did this as early as 4 years ago.
This research result released by Google in 2016 is also an end-to-end learning framework, learning from millions of examples and significantly improving the quality of translation.
The translation system not only improves the translation quality on the test data, but also supports translation between 103 languages, translating more than 140 billion words every day. Although there are still some problems, Google did achieve 100 languages.
Let’s take a look at how this Google system works.
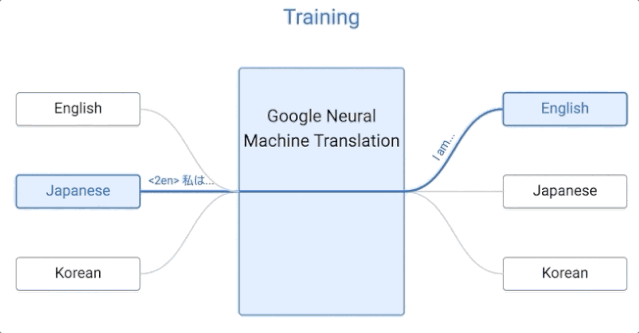
Google’s algorithm is zero-sample learning. Suppose we use Japanese, English, and Korean examples to implement a multi-language translation system, which is the same size as a single GNMT system. It shares parameters for these different language pairs. Translation between. This sharing enables the system to transfer the “translation experience” from one language pair to another.
“Facebook’s declaration of not relying on English data is also inaccurate.”
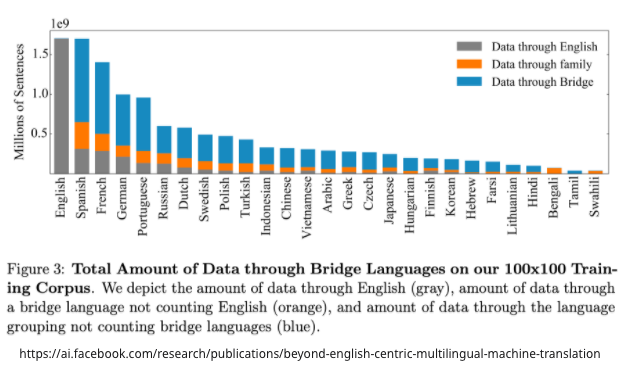
The Facebook paper chart shows that some of the data set used contains English. To say that there is no dependence on English at all, it kind of obliterates the role of English.
So far, Google has papers discussing training in 103 languages and a separate paper that does not “rely on English data.”
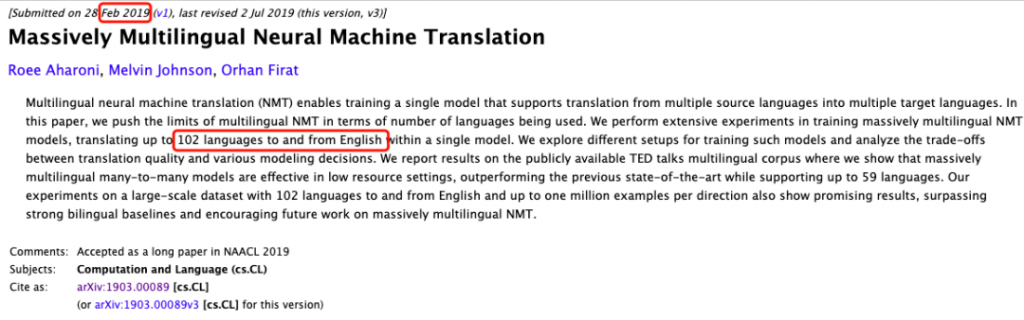
The large-scale multilingual machine translation released by Google in 2019 supports 103 languages, but the source or target language is English.
From the perspective of technical accuracy, it is indeed difficult to find a paper that can meet two requirements at the same time: it does not rely on English data and more than 100 languages.
Netizens believe that a non-misleading statement should be, “Facebook created a huge NMT data set and trained a Transformer on it.”
Regardless of whether Facebook’s statement is accurate, its model effect is indeed better than before, and related data sets and codes have been open sourced. Students with computing resources can verify it.
So, will human translation be replaced by machine translation?
Will machine translation completely replace human translation? Thinking too much!
With the continuous progress of machine translation technology, this has become the most concerned issue for more and more people, especially those in the translation industry.
This is not “underlying worry”.
Whether it is Facebook’s recently open sourced M2M-100 model, or Google’s previously released AI translation that supports 103 languages, it shows the huge possibility of machine translation replacing human translation.
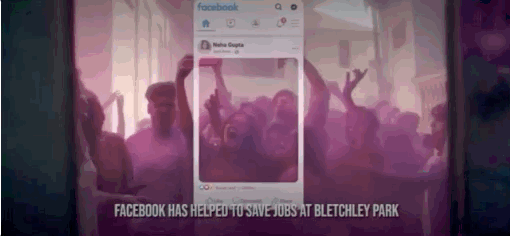
However, in terms of the current development of machine translation, it is still not realistic to completely replace human translation.
From a technical point of view, there are still many technical difficulties in machine translation that need to be overcome, such as disordered word order, inaccurate word meaning, and isolated syntactic analysis.
From the perspective of practical application, machine translation cannot achieve accurate and rapid translation in some colloquial translation scenes, scenes that require relatively high professional knowledge background, and scenes of large conversations.
Earlier, the media broke the news of many machine translation “rollover” incidents. For example, the machine translation of large-scale conferences showed a large number of unreasonable sentences, some names could not be recognized, and some daily conversations were also translated to laughter…
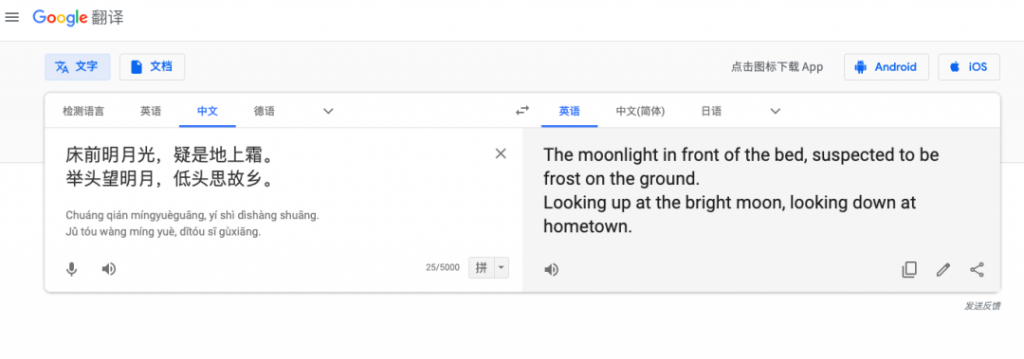
Although the performance is not so satisfactory, the rapid development of machine translation will undoubtedly eliminate a group of low-level human translators. Those human translators who can only perform “low-end” translation will undoubtedly be replaced by machine translation.
And truly high-level translators don’t need to worry about this problem at all. Even with the most advanced machine translation, there is still a big gap between the translation requirements of “faithfulness, expressiveness, and elegance”.
On the contrary, machine translation can liberate high-level translators from some mechanical and boring simple translation work, let machine translation become a tool, and spare energy to engage in more creative work .
In fact, future translators may be closer to editors and quality control experts, and are more likely to modify and polish the first draft of machine translation and literary creation.
All in all, it is a futile thing for machine translation to completely replace human translation.
AI companies like to exaggerate publicity, artificial intelligence is based on “ifelse”?
Facebook’s model that seems to replace human translation has caused a lot of discussion.
Some netizens even think that the field of machine learning is always misled by public opinion.

The research or voices of some large companies are easier to hear, and even have certain advantages in accepting papers.

Although most of the paper reviews at top conferences are now double-blind, it is easy for reviewers to judge the author’s background. For example, if the model in the paper uses thousands of TPUs, it is undoubtedly from a major manufacturer.
Large technology companies such as Google and Facebook do occupy many advantageous positions.
Some AI companies like to use these papers to exaggerate the role of AI in practice.
Moreover, press releases are sometimes written by non-researchers based on limited descriptions or abstracts, and may not have any fact-checking, leading to certain deviations.
Previously, there was a news about Uber on Twitter that attracted a lot of attention. This tweet quoted a press release, which stated: “Uber will use artificial intelligence to identify drunk passengers. The location of the car and the user’s hesitation time and other parameters are judged.”

The following sentence is written: “That’s not AI. That’s just an if statement.” It also gives the code to implement this intelligent recognition system, which requires two lines in total:

In fact, it may not be that simple.
Uber may use machine learning and fine-tune the weight of the model based on past data. It can also use wrong judgments to update the predictive model, but some AI applications are indeed not as good as in the paper.
So, have you written an artificial intelligence application based on ifelse?
Comments